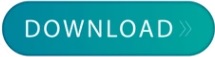
#Sun corona hole 2016 series#
calibration of sunspot number series (see Clette et al. Such an extended data set would be interesting with respect to e.g.
#Sun corona hole 2016 manual#
This trick can hardly be done by manual threshold levels adjustment since many aspects of individual image perception are difficult to formalize explicitly. Applying the trained network to a larger time interval one can create an extended data set of active regions isolated by methodology similar to original one. at some observatory or by particular astronomer. The point is that neural networks can learn a methodology of active regions isolation that was used e.g. In our opinion, investigation of neural networks with respect to segmentation of solar active regions allows some possibilities beyond the capacity of hand-engineered algorithms. We failed to find any publication relevant to neural networks usage for active regions segmentation, although the amount of available data for neural network training is more than enough. In application to solar data, Díaz Baso & Asensio Ramos ( 2018) presented deep learning techniques for enhancing SDO/HMI images, while Asensio Ramos, Requerey & Vitas ( 2017) trained a neural network to estimate horizontal velocities at the solar surface. While contrast enhancement, thresholding and geometrical considerations were massively exploited in computer vision for many years in a number of domains, more and more recent publications demonstrate that computer vision algorithms based on neural networks can provide significantly better results in object detection in comparison to conventional hand-engineered algorithms (see e.g.Chen et al. The Spatial Possibilistic Clustering Algorithm (SPOCA) requires a proper intensity normalization to apply fuzzy clustering method for image segmentation (Barra et al. Similarly, multi-thermal intensity cut is a key step in the Coronal Hole Identification via Multi-thermal Emission Recognition Algorithm (CHIMERA Garton, Gallagher & Murray 2018). Scholl & Habbal ( 2008) apply contrast enhancement in a multi-passband detection method in 171 Å, 195 Å, and 304 Å passbands. Morphological image analysis, thresholding and smoothing were applied by Henney & Harvey ( 2005) for CH detection in solar spectroheliograms. 2014) also includes thresholding at some fraction of median pixel value. The Automatic Solar Synoptic Analyzer (ASSA Hong et al. For example, Krista & Gallagher ( 2009) identify CHs using a histogram-based intensity thresholding.
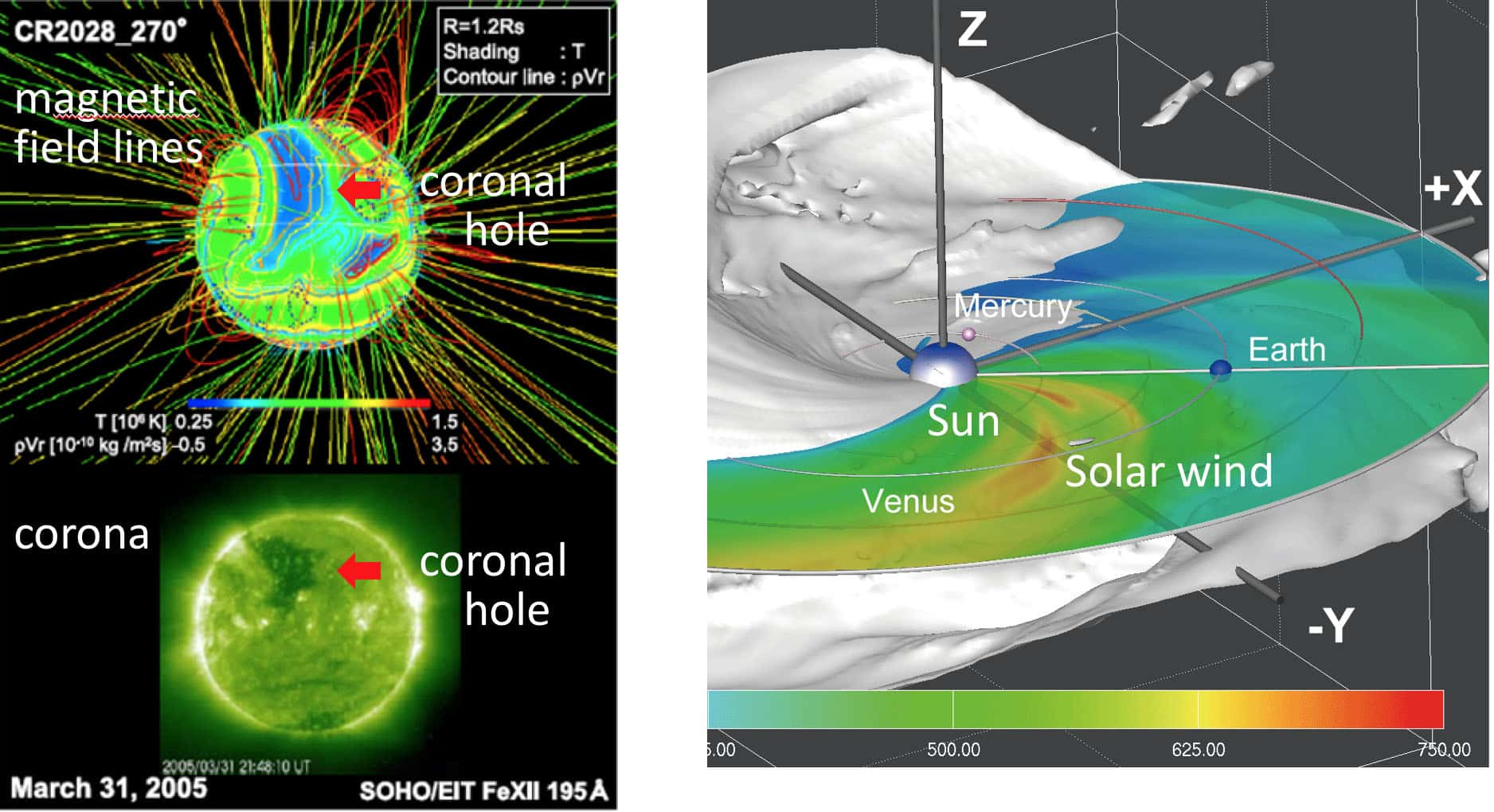
CHs have irregular profile and may occupy more than 10 per cent of visible solar hemisphere.įor segmentation of CHs one typically applies rule-based approaches that include image thresholding and region growth steps. They appear as dark regions due to their lower density and temperature in contrast to surrounding atmosphere (see Priest 2014). An accurate CHs segmentation procedure is essential for more detailed investigation of this relationship and implementation of space weather forecasting routines.ĬHs are best seen by eye in images in the 193 Å wavelength. 2009 Abramenko, Yurchyshyn & Watanabe 2009 Rotter et al.
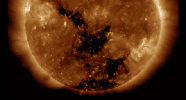
1976 Robbins, Henney & Harvey 2006 Obridko et al. Altschuler, Trotter & Orrall 1972 Krieger, Timothy & Roelof 1973) and provide an estimation of SW parameters and corresponding geomagnetic effects (e.g. They are typically associated with high speed solar wind (SW) streams (e.g. Methods: data analysis, techniques: image processing, Sun: corona, solar wind 1 INTRODUCTIONĬoronal holes (CHs) are regions of open magnetic fields in the solar corona.
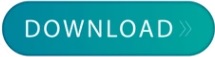